Multi-task learning (MTL) can improve the generalization performance of neural networks by sharing representations with related tasks. However, MTL is challenging in practice, as negative interactions between tasks can degrade performance. We draw inspiration from physics, deep learning theory, and sample-level weighting to analyze these complex interactions and develop intelligent task-weighting algorithms. Furthermore, we aim to apply MTL across a diverse range of fields.
Papers
PRESENTATIONS
Towards sustainable power systems: improving battery degradation forecasts with enhanced multi-task learning
Emilie Grégoire, Xia Zeng, Sam Verboven, Maitane Berecibar
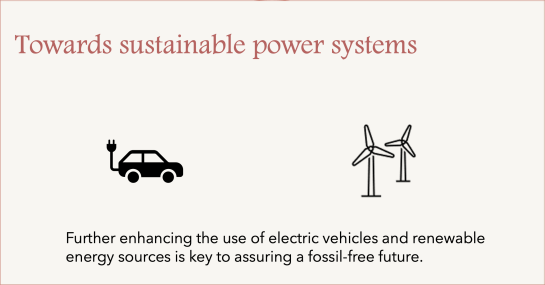